Project aims to improve accuracy of selecting resistant fish for breeding purposes
The Danish Technological Institute (DTI), a non-profit focused on high technology solutions, and Benchmark Genetics, a world leader in breeding and genetics on Atlantic salmon, tilapia, shrimp and lumpfish, are collaborating on a research and development (R&D) project that involves using artificial intelligence (AI) to count sea lice. The goal is to improve the accuracy of selecting resistant fish for breeding purposes.
Currently, to assess the degree of resistance of Atlantic salmon to sea lice, breeding companies count the number of parasites on each fish following a period of infection. But this process has challenges.
“This is a laborious and time-consuming process involving many people needed to count the lice manually,” wrote the partners in a press release. “However, this process can now change, thanks to [this new collaboration]. By taking photos of each fish and using artificial intelligence (AI) to analyze images in real-time, the work obtained an accurate number of lice infections per animal.”
The imaging technology uses a combination of a half-circular light-dome (CSS dome light HPD2-400FC) and a 5-megapixel monochrome camera to take an image of each salmon. The high-power light dome has three individual triggered colour diodes: red (622 nm), green (525 nm) and blue (470 nm). The mono-camera takes one picture at each wavelength, and then images are post-processed using the developed AI.
“It was found that this imaging system provides the user with the best visibility and contrast between the lice and the fish,” said the partners. “The algorithm uses a deep learning segmentation model based on a multiple convolutional network architecture U-net image model, initially developed for biomedical image segmentation.”
The AI model is trained to segment the sea lice and salmon. After image segmentation, the sea lice are filtered and counted. By repeating this process and automatically augmenting the image appearance in various ways, the model learns the shape and becomes robust against varying fish and lice sizes, image angles, illumination variations and so forth.
A helping hand to lend: UK aquaculture seeks to broaden its horizons
“The training dataset is created by manually marking pixels with lice in the image,” said the partners. “The model can then compare its results against the training dataset and gets trained. The bigger the training dataset, the more accurate the algorithm can detect lice. The model’s performance improvement is verified by keeping a subset of the annotated data for model validation.”
Once the new technology is implemented, Benchmark Genetics expects to gain a higher quality of the phenotypic datasets and improve the precision in selecting the best breeding candidates for resistance to sea lice.
Follow the Advocate on Twitter @GSA_Advocate
Now that you've reached the end of the article ...
… please consider supporting GSA’s mission to advance responsible seafood practices through education, advocacy and third-party assurances. The Advocate aims to document the evolution of responsible seafood practices and share the expansive knowledge of our vast network of contributors.
By becoming a Global Seafood Alliance member, you’re ensuring that all of the pre-competitive work we do through member benefits, resources and events can continue. Individual membership costs just $50 a year.
Not a GSA member? Join us.
Author
-
Responsible Seafood Advocate
[103,114,111,46,100,111,111,102,97,101,115,108,97,98,111,108,103,64,114,111,116,105,100,101]
Tagged With
Related Posts
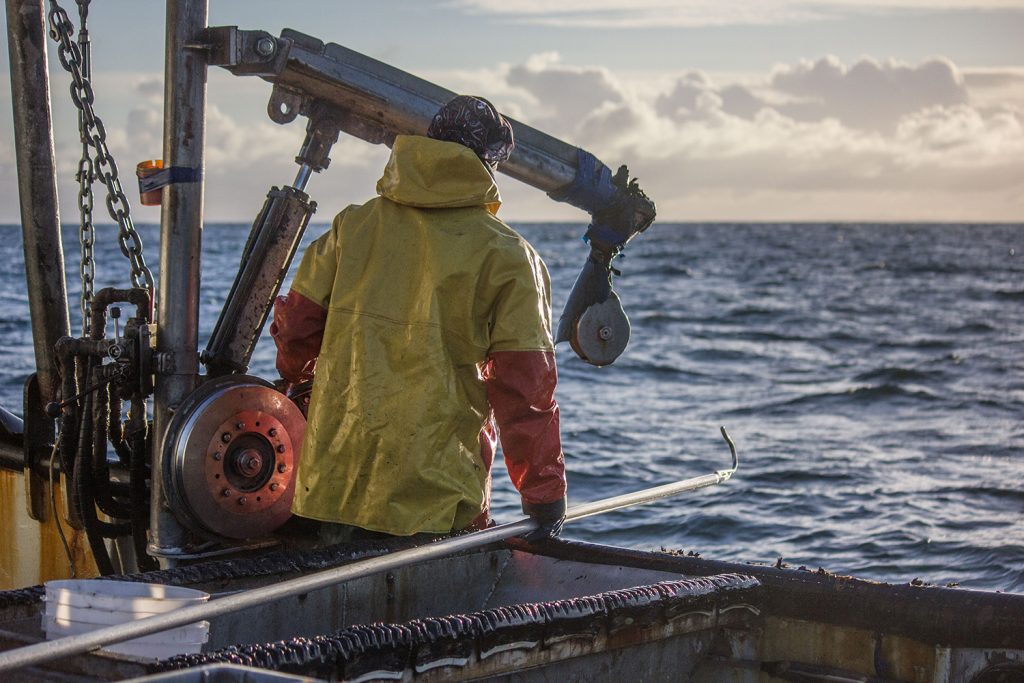
Fisheries
Artificial intelligence is already helping improve fisheries, but the trick is in training the tech
Artificial intelligence is providing valuable data to fisheries, cutting costs and the need for human review. Can the technology be perfected?
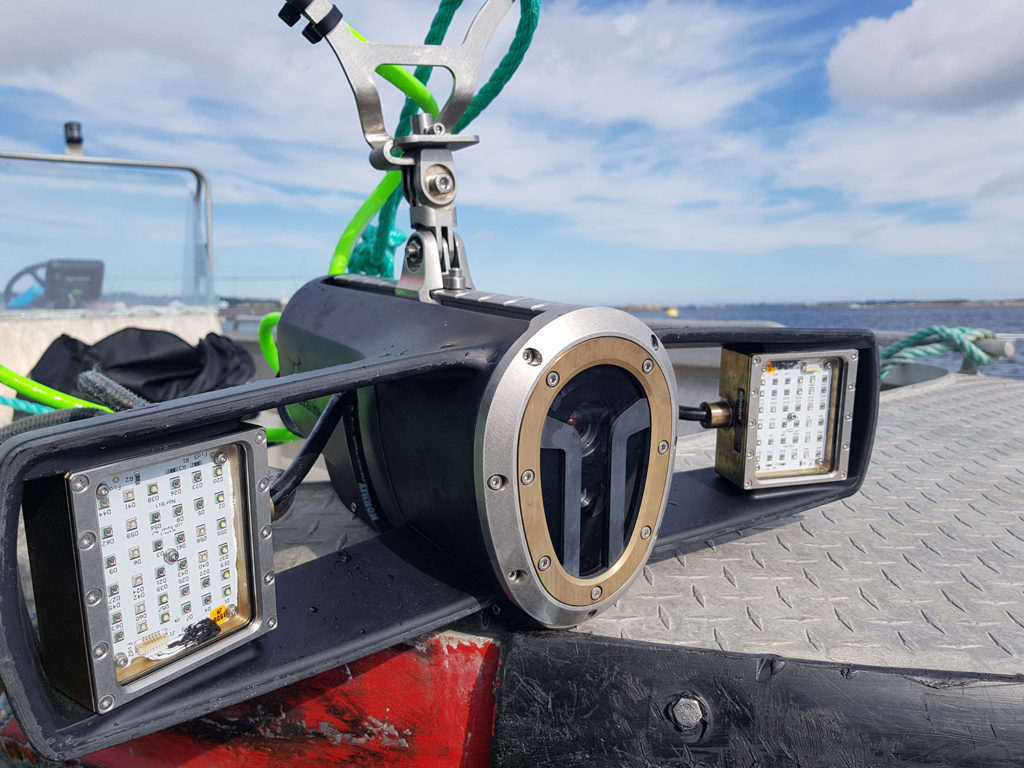
Innovation & Investment
Farmers deploy new high-tech tools against sea lice
Salmon farmers in Norway are trialing two new technologies, including one from Silicon Valley, in their ongoing battle with the persistent sea lice.
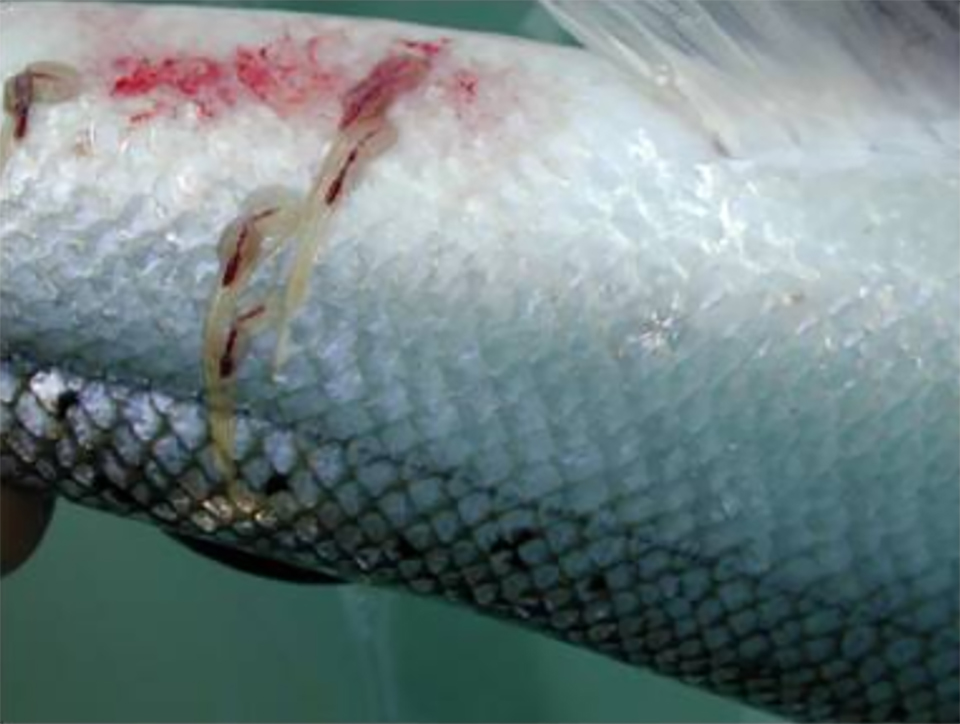
Health & Welfare
Norwegian researchers develop sea lice tracking model
An operational salmon lice model calculates sea lice infestation pressure all along the Norwegian coast in near real-time, based on a hydrodynamical ocean model and a salmon lice particle tracking model.
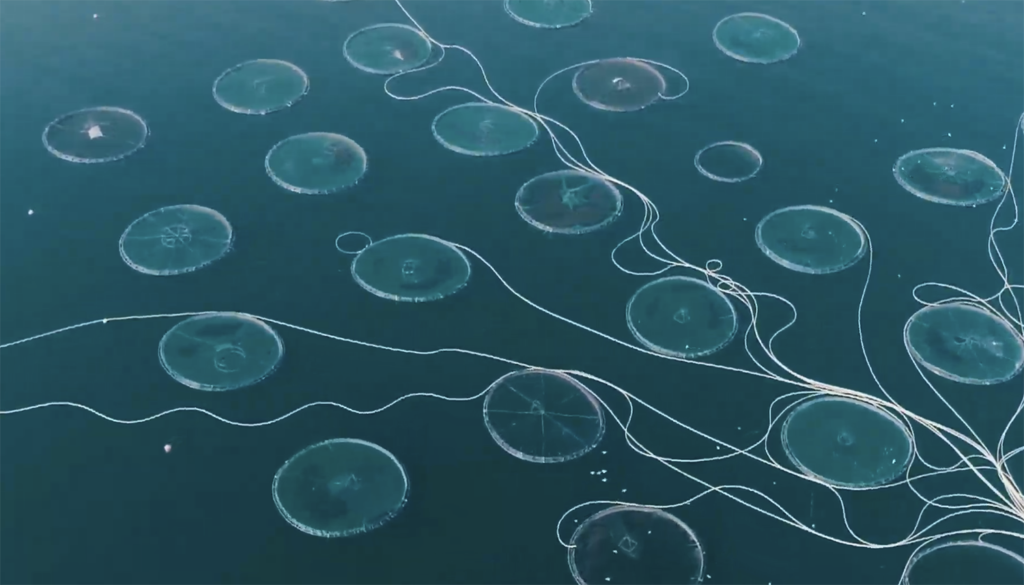
Innovation & Investment
Apps, AI and algae: Meet Hatch Blue’s fourth cohort
Hatch Blue’s fourth cohort performed their pitches online for the first time, showcasing a spectrum of aquaculture product and service businesses.